By Ben Polidore, Fangyi Li and Zhixian Chen
Market, let prices be efficient, but not yet. —Trader’s prayer
When an order has higher-than-expected costs, it is interesting to attribute those costs to various factors to make the post-trade analysis more actionable. In ITG’s post-trade cost models, we have an “others’ impact” factor, meaning impact from other market participants on the same side as your order. The idea is that when you control for your own liquidity demand, you can infer the imbalance of demand from the rest of the market. If the others’ impact is unfavorable, this means there is an imbalance of participants trading the same security on the same side—e.g., more buyers when you are buying.
Of course, the first question you ask when presented with such a statistic is why other participants were trading at the same time. Coincidence? What if others’ impact is systemic? Same investment process? Could be. Or it could be that others’ impact is a consequence, not a coincidence, of your order—that your order is creating others’ impact. This is information leakage.
Information leakage is a major concern to asset managers. In a recent ITG survey of 82 buy-side traders, 35% of respondents claimed that information leakage represents the majority of their transaction costs. Unfortunately, information leakage is hard to measure and harder to attribute to specific venues and behaviors.
In this paper, we document our proprietary approach to controlled measurement of information leakage in dark pools. We demonstrate that the standard approach used by most brokers—ranking dark pools using a post-trade reversion (“adverse selection”) benchmark—does a poor job of predicting the true costs associated with routing to a specific dark pool. In contrast, our new methodology draws a line between dark pool information content and parent order performance, and it tells a very different story from that told by adverse selection.
ADVERSE SELECTION VS. INFORMATION LEAKAGE
When you think about adverse selection, it’s best to focus on the word “selection.” Adverse selection, whether in insurance or limit order placement, occurs when a counterparty with better short-term information selects your standing offer, creating a loss. Your limit prices should reflect this risk.
There are two key differences between adverse selection and information leakage:
1. Unlike information leakage, adverse selection is not caused by your order.
2. Adverse selection is measured on fills, whereas information leakage is really about the parent order and may not require fills.
The way you measure adverse selection is to look at the price after you have been selected. If the future price is more favorable (meaning the price of a buy went down), then you regret the trade. Conversely, if the future price is worse (meaning the price of a buy went up), this is deemed a positive outcome. Herein lies a problem: When your trading activity is causing prices to move away, this activity is rewarded with a positive adverse selection benchmark. This is especially costly if the information-leaking fill happens early in the life of an order.
Measuring information leakage directly at the parent order level is a more economically interesting prospect, but it requires a large amount of data and direct technology support from the algorithm itself.
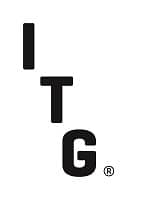