UBS has developed a state-of-the-art machine learning (ML) technique to search for patterns in data that skew the outcome of passive order placement on equities venues towards higher fill rates, lower information leakage, lower market impact and lower execution fees.
When considering which venue to select for posting passive liquidity, it is important to get a granular understanding of how your firm’s trading profile interacts with each venue according to a range of metrics. Marketing data received directly from venues around execution performance typically reflects the average experience of the whole venue. This will inevitably be weighted towards how certain participants perform, based on the context in which those participants trade. That performance will not necessarily hold true for each firm’s unique experience when trading on that venue.
“The properties of any one individual venue could affect participants in the market differently based on the timing and context of their trading,” says John Fruen, head of market structure and liquidity strategy EMEA, UBS. “Therefore, it is more important to rely on an individual firm’s experience than to rely on the idea that a venue has inherent properties which can be leveraged, without knowing whether they fully fit with the trading firm’s order flow. Moreover, understanding the context in which an order is being placed creates the opportunity to utilise differences in venue experience at the most opportune moments.”
Estimating execution quality in passive trading, has historically focused on measures such as speed or likelihood of an order being filled. Whilst both fill ratios and mark-outs are important factors, they ignore any potential information leakage and opportunity cost of unfilled orders.
“Average daily volume [ADV] was often used in legacy models,” says Fruen. “The issue with that is it creates a ‘chicken and egg’ problem – if you assume a venue has poor trading performance because it has no volume, you’ll never send order flow there and never experience what that venue is like.”
Using machine learning to improve trading
Not only is that use of ADV restrictive, it also assumes that venues are static, and that is far from true. To reflect the need for a more dynamic approach to smart order routing (SOR), UBS has developed a machine learning model to better support routing decisions – initially for passive order flow. It is looking for patterns which correlate with better execution outcomes – higher fill rates, reduced market impact and information leakage, as well as lower fees – on lit equity venues.
With UBS’s approach, the innovation is the use of the Bayesian Decision Tree model, which offers transparency over the way outputs are selected, and fits the necessary characteristics for equity trading. It is low latency and can consider both parent order and orderbook information when making a decision on order placement, based on the differences between the characteristics of each relevant venue in different contexts.
A structural advantage of employing machine learning tools is the adaptability it shows to changing market circumstances, adapting in real-time to reflect the changing properties of a trading venue.
“Given properties might change relatively quickly, based on new members coming on board, or order flow decisions, it pays to continually explore and adapt to those changes,” notes Fruen.
Traditional approaches can be limited by their reliance on a daily measure such as ADV, where the UBS model uses Thompson Sampling to balance the need to explore venues to find the best execution experience against using historical data to determine which venue is likely to perform best in the current market conditions.
“The Thompson Sampling methodology offers a way for us to optimally manage the trade-off between capitalising our knowledge [drawn from historical experience] and exploring potentially new valuable placement strategies,” says Giuseppe Nuti, UBS’s head of Agency Quantitative Research.
Seizing the opportunity
In the US, the A/B trading scenario on the use of UBS’s Machine Learning Smart Order Router produced some interesting results, bearing out the case that habitual order routing patterns have room for improvement.
One of the key advantages of this approach is in its ability to explore new order types and quickly determine the market and order context that may be most appropriate for leveraging the new offering. For example, it interacted very positively with the D-Limit order type offered by the Investor Exchange (IEX). The D-Limit will make an order one tick more passive if IEX thinks the market is likely to move towards it based on an order book signal.
“IEX is trying to limit adverse selection, defined as trading only when the probability of the market trading through our limit price is high,” says Nuti. “Instinctively, the benefit of this order type ought to be more pronounced when queue sizes are large, since the signal of a crumbling quote, at the heart of IEX’s offering, will be clearer for larger queues. Yet we found that the model learned to leverage the IEX D-limit more for smaller queues based on two interesting, and not necessarily obvious, observations. Firstly, small queues will be subject to more frequent tick changes, i.e. leverage the inherent insurance in the IEX D-limit when you get to use it more frequently.
Secondly, choosing IEX for a limit order joining a large queue will, by definition, forego being on other venues, which may offer a higher rate of natural queue depletion (and thus a higher likelihood of a fill not resulting in adverse selection).
A further example of some of the interesting behaviours observed by this approach is on the use of inverted venues – those where the aggressor gets paid to take liquidity. Various studies have shown that information leakage for posting tends to be quite high, as the market interprets the order as effectively narrowing the spread.
In essence, inverted venues offer an improved fill probability, paid for in fees and information leakage. Overall, UBS believes that the market has adjusted to the various fee structures, offering little discernible advantage for posting on inverted venues under normal circumstances. Since the value of routing to an inverted exchange increases with the width of the spread, as conditions in the market present wider prices, it becomes increasingly more valuable to leverage inverted venues. This is illustrated above (where the spread ratio represents the ratio of the spread at limit order insert over the average spread for that stock for the day): the wider the stock is versus its average spread, the more we can leverage inverted venues.
The context-aware ML technique is embedded in UBS’s US and EMEA cash equities SOR, and the firm is further looking to use it in dark order routing to find midpoint liquidity, and when crossing the spread.
To learn more about our in-house developed machine learning techniques, please contact the UBS trading desk.
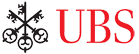