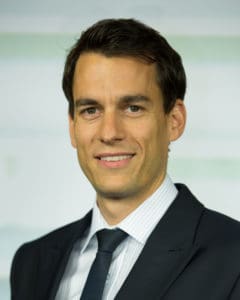
Christian Gressel, global head of electronic sales, UBS
What developments and trends are you seeing from your clients’ liquidity requirements?
Christian Gressel: We are seeing clients taking two approaches, with a majority expecting the broker to manage liquidity and policing this on outcomes, whilst a minority look to be more active in this selection process.
Typically, clients assess us on the overall performance of our executions and allow us to make routing decisions based on the full spectrum of enabled liquidity sources. One important driving factor for this of course is regulation and we do see a strong need from clients to fulfil best execution requirements and hence need access to multiple venues for their algorithmic executions. They typically want to know, post trade, which venues they’ve executed on, have a high level of transparency on child order prices and very often have their data send to a third party for an independent assessment.
The second group of clients who actively chose a specific liquidity profile either select to execute against UBS only or have some variation of our full offering. The former strongly believe in the quality of UBS principal liquidity and the benefit this source has on the outcome of the parent order by limiting market impact of individual child orders.
They are mostly non-European clients whose regulatory pressure to add multiple sources in the mix is perhaps not as sharp as it is for their European peers and who purely care about the overall outcome. Then there are a smaller set of clients who have special requirements like wanting a stream of third-party liquidity sources as they might receive a streaming price from UBS directly or have a particular venue in mind they want to execute on.
What should clients be aware of when having liquidity discussions with their algo providers?
CG: First of all, more is not always better when it comes to liquidity. There is a limited volume of real liquidity in the market and we found that adding a growing number of electronic communications networks (ECNs) often means little more than adding a new route to the same pool but at a different ECN; sometimes even leading to negative effects like increasing rejection rates due to hitting the same liquidity via multiple points or increasing impact as liquidity gets immediately recycled in the market.
Secondly, in FX you deal with very different types of liquidity, ranging from firm on the CME via the futures central limit order book to various degrees of how last look and cancel priorities are applied. Being able to correctly compare prices between these different sources has a major impact on your overall trading cost. This is mostly dealt with by trying to homogenise the pools as much as possible by setting rules or minimum requirements for participating liquidity providers on maximum allowable rejection rates and minimum holding periods. This gets them to the point where prices between ECNs can be compared on a like for like basis but we found it limits the available liquidity and access to aggressive pricing overall.
How is UBS dealing with these differences in the FX microstructure?
CG: UBS has taken a different approach to other providers. While we do of course care about the quality of market makers in our different pools we’ve concentrated on building a mix of complementary liquidity sources that consists of the primaries, a small number of ECNs, the CME futures contracts and UBS principal liquidity. However, the real differentiator is in how we value prices we see from different sources in order make the correct routing decisions.
We continuously measure rejection rates and the cost of resubmissions these invariably create and take this into account when calculating the real value of each price in order to create an equal basis for comparison. This means we don’t have to limit the liquidity we’re looking at but understand how much price improvement we need to see from a venue with higher rejections than from one with lower ones. Interestingly, once you factor in these rejection rates and resubmission costs, your cost of trading is very similar across different venues irrespective of the headline spreads shown.
Ultimately liquidity providers will need to be able to make markets profitably and face a trade-off between how tight they want to show their spreads versus how high their rejection rates are. As a consumer of liquidity understanding how they get paid and being able to execute accordingly is the important thing.
What has been the effect of this approach for clients using your algorithms?
CG: Our principal trading desk have been using this technology for their hedging needs on external markets and have seen a reduction in execution cost by approximately one third in combination with steadily increasing fill ratios for nearly a year before we took the decision to use the same smart order router for client algorithms. Clients have since benefitted from the same reduced trading cost and improved fill rates for urgent orders with this technology via ORCA Direct.
For our schedule-based algorithms, however, we’ve already seen that clients using UBS liquidity benefitted from spread compression in combination with very little market impact. Our biggest concern was hence naturally whether the benefit of additional liquidity was going to be outweighed by creating bigger impact. A year on from underpinning our algorithms with the new smart order router we can see that this technology has further improved the performance of our algorithms measured by arrival price benchmark.
How has UBS developed its algorithm offering to reflect market changes and how will this evolve in the future?
CG: Aside from these major improvements to how we deal with the microstructure of the FX market, we are also improving the macro side of our algorithmic strategies. One area of continuous development are our quant parameters. These are the inputs that feed our algorithms with the necessary market information.
We look at a long list of different parameters ranging from observed spreads, market volatility, price development to how long we need to see a price until it’s deemed a valid price. Like a trader, our algorithms will be comparing these inputs at any given time with their “normal” values and take a calculated trading decision in order to achieve the best possible outcome within the wider framework set by the user. The better and more accurate (for a specific point in time) these inputs are, the better the outcomes and overall performance will be.
A second area of quant development is the implementation of a multi-factor model consisting of close to 100 different values our algorithms look at when predicting upcoming price movements. These will range from economic data to the price action observed in stock market indices and the futures contract of the corresponding currency pair and will further improve our ability to make trading decisions.
One of these factors will be the composition of an order book and possible imbalances we see in its current state. It’s easy to see how a totally skewed order book when you’re trying to execute a relatively small order leads to a clear choice between trading immediately or waiting to be filled passively. But when you’re dealing with various degrees of imbalance in combination with your order size and many other influencing factors at the same time it becomes clear that advanced models and machine learning will have a growing influence on how algorithms execute. We will shortly be releasing upgrades to our offering under the hood that benefit from this work.
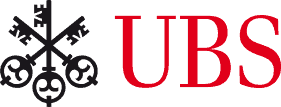